Learning Analytics (LA)
Advanced LA is more than statistics or an AI-technique. It is the engine of modern learning ecosystems, providing a process to select, gather and analyse more detailed data on proficiencies. Its purpose is to optimise learning and training, for example by recommending the next learning or retention exercises in the most effective way, not only for one training phase, but – ultimately – taking the full training and operational life cycle into account.
It relies on AI to create and improve predictive models of learning and retention.
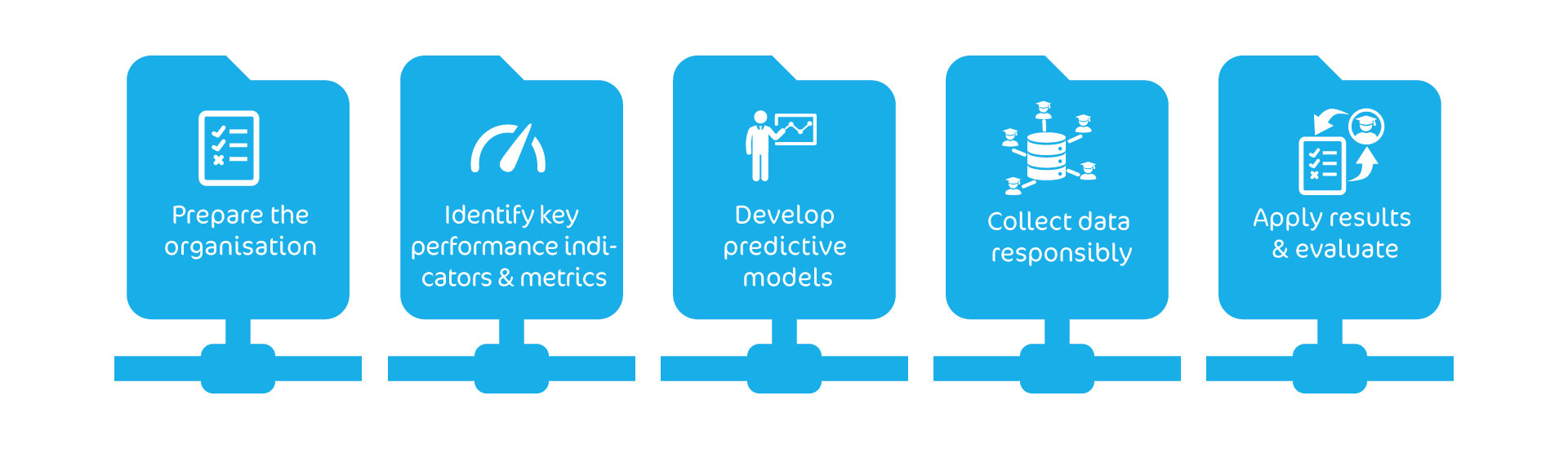
LA requires development or consideration of the following:
Prepare the training and operational organisation for a data-intensive approach
- LA may start by using subjective instructor assessments or self-ratings that are linked to the trained competencies. This often requires more detailed observations or assessments which in turn requires an easy to use scoring app.
- More powerful LA will require automatic logging of data from operational systems or training media.
- Measures need to be taken to ensure appropriate security and privacy levels.
- The data-intensive approach may require a learning ecosystem data infrastructure.
- The disruptive changes in the training regime require a small steps roadmap and careful change management.
Define metrics for (tactical) proficiencies and learning or retention indicators
- Development of learning and retention metrics is a continuous process and a multidisciplinary undertaking.
- The starting point is to develop competency-related performance indicators that may be observable by instructors or automatically measured.
- Complex metrics requiring automatic data logging from live systems or training media may be added gradually to the LA system, starting with the most relevant as well as the low-hanging fruit measures.
Select suitable techniques to develop predictive models for learning or retention
- Initial data and data-analysis may support instructors to fine-tune assessments and find root causes of issues.
- When sufficient data has been gathered, AI techniques may be used to develop predictive models of learning or retention. Based on these models, a best-fitting next exercise can be selected and scheduled for each individual to ensure the right type and level of exercise is provided in a suitable time frame.
Implement data logging protocols
- Ensure live systems and training media (manufacturers) allow for data logging.
- Ensure data is well-protected in terms of security and privacy.
- For example, for qualified professionals, the owner of data may be the professional, while only certain high-level metrics are provided to superiors, for example to be informed on readiness levels.
Apply predictive models continuously to recommend optimal next exercises and scheduling
Using predictive models, a best-fitting next exercise can be selected and scheduled for each individual to ensure the right type and level of exercise is provided in a suitable time frame. With data from every new exercise, the predictive model will adjust its prediction and recommendation continuously. Naturally, scheduling needs to be optimised taking the needs of other students or team members and organisational constraints into account.